
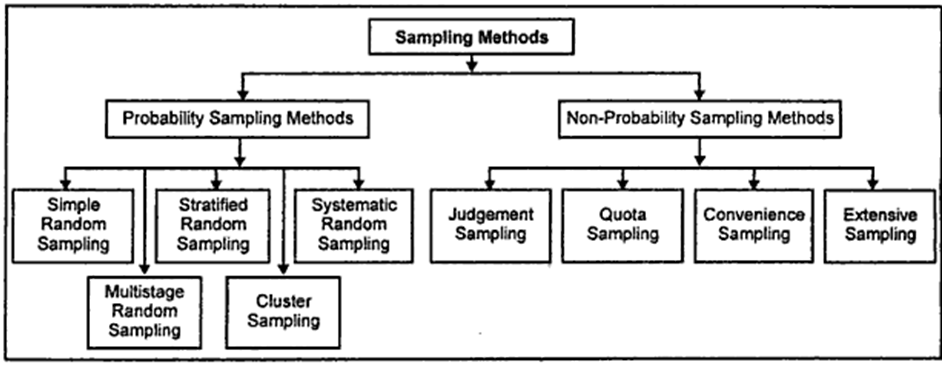
A crude measure of population prevalence is the fraction of positive tests at any given date. It is widely accepted that a large fraction of COVID-19 cases go undetected.
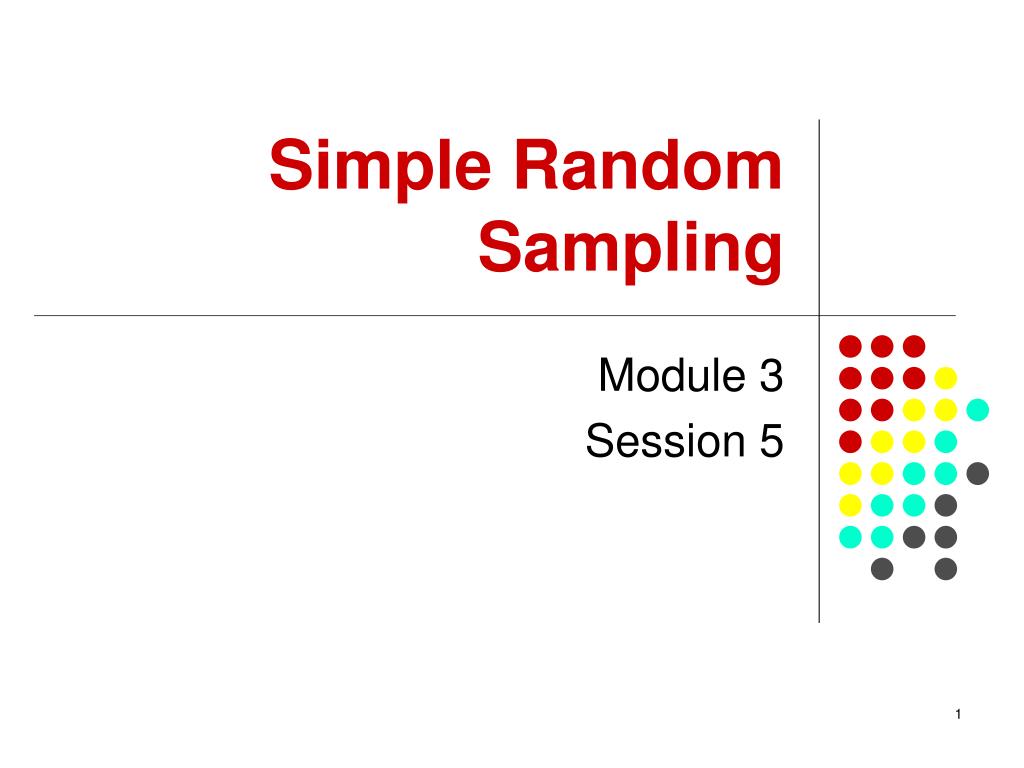
Sample pooling should be considered in COVID-19 prevalence estimation efforts. Sample pooling can be particularly beneficial when the test has imperfect specificity by providing more accurate estimates of the prevalence than an equal number of individual-level tests. Even when the true prevalence is as high as 10% it can be appropriate to pool up to 15 samples. In low-prevalence populations, it is theoretically possible to pool hundreds of samples with only marginal loss of precision.
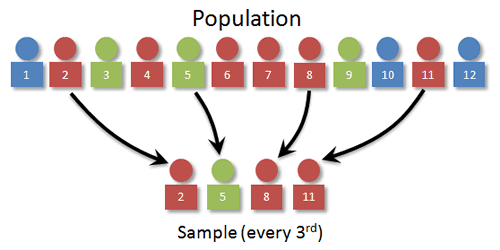
Sample pooling can greatly reduce the total number of tests required for prevalence estimation. Here I use simulations to explore how experiment sample size and degrees of sample pooling impact precision of prevalence estimates and potential for minimizing the total number of tests required to get individual-level diagnostic results. MethodsĮstimates of the true prevalence of COVID-19 in a population can be made by random sampling and pooling of RT-PCR tests. However, this fraction depends heavily on the sampling intensity and the various test criteria used in different jurisdictions, and many sources indicate that a large fraction of cases tend to go undetected. The number of confirmed COVID-19 cases divided by population size is used as a coarse measurement for the burden of disease in a population.
